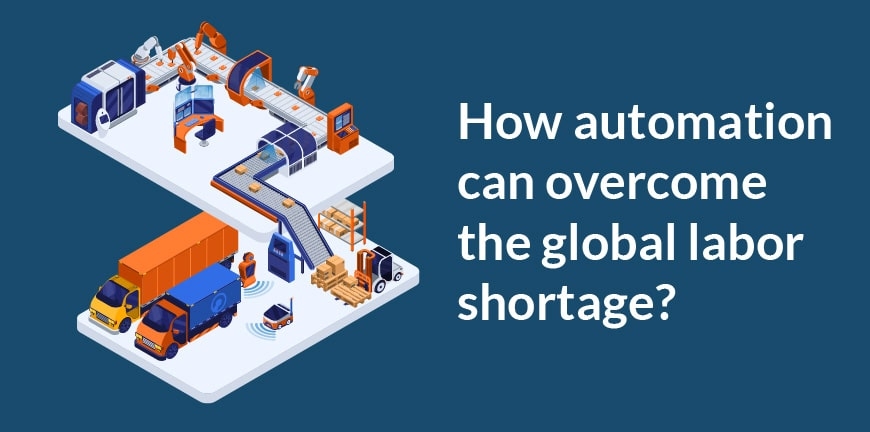
How Automation Can Overcome the Global Labor Shortage
17/07/2023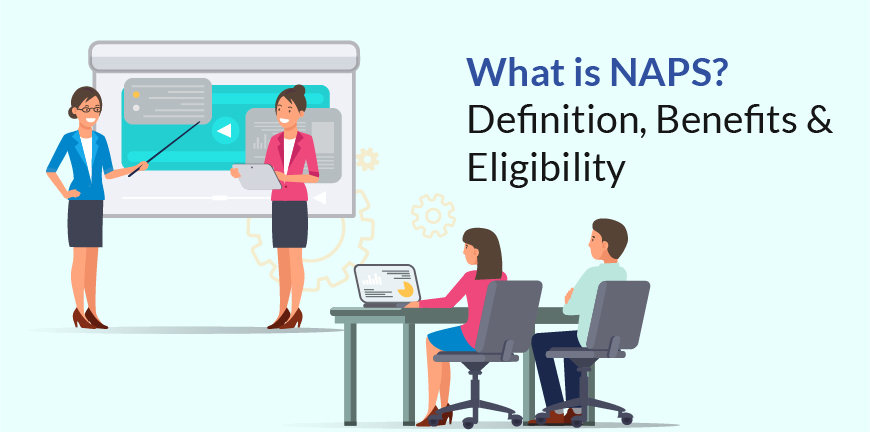
What is NAPS Scheme? Definition, Benefits & Eligibility
18/07/2023Everyone knows J.A.R.V.I.S, the super-intelligent AI behind Iron Man’s Suit. Well, we are well on our way to transforming technology using AI that is just as powerful in the years. For starters, the fourth iteration of ChatGPT, the generative AI everyone is talking about, is already here and the fifth is already here.
It can write blogs, compose songs and poetry, and even draw up digital transformation strategies for startups. It has ignited the human consciousness in ways previously though impossible with its intelligence, and to a certain extent, creativity. AI, and not just large language models like ChatGPT-4, are disrupting healthcare too, as we speak.
In fact, the advancements are so rapid that regulatory bodies may be unable to keep up with it. The faster processing and the wealth of information AI systems have gathered over the Internet are very strong reasons for it. The challenges that lie ahead of AI before it can truly revolutionize healthcare are many. Some of them are outlined here in this article.
1. Siloed and legacy infrastructure
The siloed and legacy infrastructure in a hospital exists because of the different departments it has and the different vendors that the hospital must work with, each working in a different area. In cases of vendor lock-in a migration from siloed or legacy infrastructure becomes virtually impossible.
Also, these silos are spread across several countries and without any kind of integration between them, it would be difficult to introduce AI or IoT into these healthcare institutions, transforming them into ‘smart hospitals.’
Also, there is a lot of legacy infrastructure such as older imaging devices and testing tools that may not be updated to work with new-generation AI. These devices may be also too old and may lack IT/OT (information technology converging with operational technology) integration as well, which is becoming very necessary for advancement in healthcare management.
2. Lack of stakeholder involvement
To revolutionize healthcare with AI, every stakeholder needs to be a part of this game-changing technology. This includes not just decision-makers who understand the impact of AI and how it can improve patient outcomes, but also doctors and caregivers who need to trust and embrace this new technology in everything they do.
Any mistrust or lack of knowledge of how these systems work could actually lead to negative outcomes for the patients. An example of this happening could be the use of AI-driven pathological assistants that help with the diagnosis of a condition. These tools make use of advanced imaging and pattern recognition to diagnose certain conditions.
The algorithm is intelligent enough to suggest possibilities and can greatly help prevent some errors in imaging and initial interpretation. On the one hand, a doctor may not want to use this system as he doesn’t trust it. This is not advisable, given the wealth of information they have at their fingertips now, with the application of AI.
On the other hand, doctors must also not trust every interpretation that the AI makes. Doctors must remember that these merely help in arriving at a decision, and that they do not make the decisions for you. For this, there must not only be trust in the AI but active participation from doctors and caregivers to further improve the quality of healthcare.
3. Privacy of patient data
Generally, AI needs a large amount of data to work with, for the sake of analysis and to draw meaningful insights. In healthcare, this translates to patient data, which is considered highly sensitive to share and use. This has raised concerns around privacy in several countries around the world.
And despite regulations, there have been instances of data sharing not being discussed with patients and the annexation of data from one jurisdiction to another. DeepMind, a machine learning company owned by Alphabet Inc., partnered with NHS in UK to transfer patient information to assist with developing treatment for acute kidney injury.
The transfer of information was not discussed adequately with patients and later this information was transferred from UK offices to the US for more detailed analysis.
Also, it is an accepted fact that several genetics testing companies are selling customer data to pharma and biotech firms, on the pretext of developing medicines based on them.
If AI must be well accepted in the healthcare and remain an integral part of it, issues such as this must be addressed. And for this, the regulatory authorities must step in and start governing how the sharing of such information happens between companies.
4. Input Bias leads to Output Bias
Any biases in the input can affect the output too Bias in the data is another problem with AI. All AI is trained on some data. If the data used to train the AI is biased in anyway, then it will reflect in the decision making of the AI.
This is known to affect certain minority communities a lot more than others. The biases that generally exist are racial bias, gender bias, socioeconomic bias, linguistic bias etc. When it comes to healthcare, other biases can also exist such as the bias when it comes to suggesting a means of treatment or even coming to a diagnosis.
An example of such bias is in a field such as radiology where the AI can make an incorrect diagnosis based on a scan and incorrectly classify the patient as having a condition, when he/she doesn’t.
Another example is that of AI being unable to draw conclusions in a group of patients when asked to identify Alzheimer’s Disease based on certain inputs received from the patients. It classified certain patients as having Alzheimer’s and others as not having Alzheimer’s and then created a third group which was inconclusive.
5. Lack of transparency
AI operations are not visible in most cases in healthcare. It is difficult to understand how the AI arrived at a particular decision because most AI in healthcare are meant to be black boxes that are highly secure because they hold sensitive patient data. And there is no workaround for this problem generally as it is implicit, and by design.
Healthcare providers who want to check for biases or inaccuracies in the AI are not able to do so because of this. An example of such AI in action is that of Watson, the supercomputer from IBM that gave unsafe procedural recommendations for doctors in oncology in 2018.
Is there a solution? Yes, healthcare providers could try and use what is referred to as Responsible AI or Explainable AI. Such AI, as the name suggests, offers explanations for why it reached certain decisions.
In this case, the AI is open for continuous development, and has a moral ground on which it is based and stands by it. The fact that it offers explanations for the decisions it makes offers more flexibility and transparency to doctors.
An example of this is the AI developed by IBM in collaboration with Highmark Health, the second largest integrated health delivery network in the US, in 2021. It allowed for greater transparency in monitoring the AI and the decisions it made in addition to several other advantages like eliminating data siloes and providing a single trusted data source.
6. Regulation and governance
There is a lack of proper regulation and governance for AI in healthcare. The EU AI Act is the first major law of its kind to be passed by a regulator anywhere, amended as recently as 14 June 2023, that takes into account most current capabilities of AI. There are three types of AI risks that it takes into consideration:
Unacceptable risk: This includes applications that are banned, which includes social scoring systems that bring about inequality among the people.
High-risk: This includes applications that have a degree of risk, but can be distributed or used, provided they are governed by legal requirements.
Low-risk, with transparency obligations: This includes AI that is low on risk, but whose providers are obligated to share information with the EU.
Acceptable risk: This includes all AI applications that are not banned or high-risk and are generally safe to use by the public. Examples include ChatGPT-4, the widely used generative AI tool.
An example of a high-risk AI application in healthcare are the safety components or regulators of healthcare devices and these must be subject to third party assessments. Another example could be an asylum management system in mental healthcare.
Under the new law, there is an obligation the owners of the AI application have to notify humans that they are interacting with an AI system, if they are not aware. An ultrarealistic voice-driven AI system for patient support might fall in this category. They are also required to inform customers if any biometric-based recognition or any kind of intelligent categorization is being applied to them.
An interesting point here is that the law does accommodate Blackbox AI and allows it to operate with a certain degree of transparency. It however requires any AI system, even the ones used in healthcare to be fully compliant with all requirements of the EU Act.
The challenge here is that this is the first act of its kind to consider current capabilities of the AI, but AI itself, especially those based on large language models or generative AI continues to grow at a very rapid pace. This means that some application of AI for healthcare may not be governed, and others may not be fully compliant, exposing patients to possible risks.
Closing words
Aye, aye, AI, but not quiet. The journey of AI in healthcare is still in its early stages and there’s a lot more it can do. But AI is not the captain of the ship, it’s just the wheel.
The healthcare researcher, doctor or caregiver is. Until this transfer of power plays out smoothly as it does between the first mate and the captain of a ship and benefits every patient on board, AI will face challenges from regulatory bodies and might even pose a risk. But when used with vigilance and care, there is nothing more spectacular or empowering care and research than AI for healthcare.