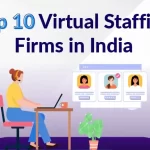
Top 10 Virtual Staffing Firms in India
15/02/2025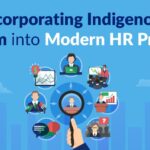
Integrating Indigenous Wisdom into Modern HR Strategies
15/02/2025“Last month, the employee turnover rate was about 20%.” When an HR manager tells the CEO of such a development, the news is not good. Guaranteed that’s true, but at least it is an actionable insight. And we must thank HR analytics for that. The growth in data analytics, and particularly HR analytics in the last decade, has ushered in a wealth of insights for businesses that can rely on them for strategic talent acquisition and workforce management.
Have Indian businesses fully tapped into HR analytics yet? How many companies learn from the interactions they have with talent, vendors, management and others? If people are indeed the most valuable resource, then companies need to justify this by investigating a bit more with HR or people analytics.
The Indian HR analytics market is growing at a CAGR of 14.3% up to 2030, which points to increased adoption in India too. The trend of smaller companies following suit does not hold good here though, with mostly mid-sized and larger companies being the top users of HR analytics. Smaller businesses may face challenges in implementing HR analytics due to limited resources and technical expertise.
What are the Types of HR Data and Classification?
1. Employee Information
Generally nominal data, both unique and non-unique, except for age which is continuous.
2. Performance Data
Can be both qualitative and quantitative. Examples of quantitative data includes the number of hours worked, project TAT, etc. while qualitative data includes candidate feedback.
3. Compensation Data
It is continuous and quantitative except for commissions and bonuses that are quantitative and discreet and benefits which are qualitative in nature generally.
4. Recruitment Data
It is both quantitative and qualitative in nature, with time-to-hire, cost per hire and number of applicants being quantitative and interview notes, candidate feedback, and notes based on resumes being qualitative.
5. Training Data
Actual scores and completion rates are quantitative but there are a lot of qualitative aspects also such as feedback from trainees and observations about the experience through interviews and focus groups.
6. Engagement Data
Generally qualitative in nature, though scores can be assigned, and a net promoter score also maintained.
7. Diversity Data
Disability and ethnicity data can be classified as nominal data and a lot of qualitative observations too.
What are the Types of HR Analytics?
HR analytics can be classified into four types based on what they help accomplish and what methods they use to get it done. The four types of HR analytics are:
1. Descriptive HR analytics – What happened?
Using statistical data, it explains and summarizes what has happened already. It does not make any predictions for the future. A couple of examples of descriptive analytics in action in HR are:
- Analyzing the average number of paid time offs availed by employees in a year.
- Comparing attrition levels of employees over the past 5 years.
2. Diagnostic HR analytics – Why did it happen?
Diagnostic analytics is based on the same data as descriptive analytics but goes one step further and gives reasons for what happened. It identifies the patterns and anomalies within the data and then studies them further to understand what factors could be attributed to them. Applying diagnostic analysis to the same two processes described earlier, we can find:
- Why have employees in a certain demographic used more paid time-offs?
- Why has there been an increase in attrition over the past two years?
3. Predictive HR Analytics – What will happen?
It categorizes past and present data to isolate patterns and anomalies in them and develops a model to predict the future based on them. In the process, the analytical model that is built to predict the future is then evaluated by applying new data to it. Let us look at two examples of predictive analytics in HR:
- Predict the average absenteeism rate of employees in the next month based on input for that month over the past five years.
- Predict what channels and locations must be targeted for recruiting candidates with a particular skill.
4. Prescriptive HR Analytics – How can we make it happen?
Prescriptive analytics takes predictive analytics further and analyses why something happened and what corrective measures to take to improve it further, hence the name “prescriptive”. Let us revisit the same two examples for predictive analytics and see how prescriptive analytics will modify them.
- Predict the average absenteeism of employees in the next month based on inputs for the given month over the past five years, why it is so, and how to reduce absenteeism by 25% in the next month.
- Predict what channels and what locations will provide candidates with a particular skill, why they do so, and how the messaging can be tweaked to improve recruitment in these zones.
Our Recommendations to Generate Actionable Insights
- Employee Information such as leaves taken and other qualitative input received from them can be used decisively. Predictive analytics can be used to predict future rates of absenteeism among employees. Prescriptive analytics can be used to suggest wellness programs and other initiatives that can improve attendance among employees.
- Look at project completion rates and the average turnaround time and feedback received. Use diagnostic analytics to understand why this has happened and develop suitable training and support programs to benefit the employees who are not performing well.
- Look at compensation data and see how changes in the salary structure could lead to attrition using predictive analytics. Suggest a corrective course of action, such as suitable incentivization, monetary or non-monetary, that can prevent this from happening.
- Look at the recruitment data such as the channels used for sourcing. Make use of descriptive analytics and look at the channels that have been most successful in the past. If the management has new hiring needs, then use predictive analytics to understand the effectiveness of current sourcing channels.
- Look into engagement data and apply predictive analytics to understand the reasons for low engagement and attrition. Then use prescriptive analytics to understand which solutions such as rewards and recognition, paid leaves etc. is most suitable to tackle the problem and increase employee engagement.
Our Recommendations to Further HR Analytics Adoption in India
The growth of HR analytics in India embraces all sectors. But there are differences across sectors which have not been clearly investigated. Some sectors have adopted HR analytics heavily while others have not. Based on one study on the prevalence of HR analytics and the popularity of HR analytics tools and the reviews it cites, we arrived at some recommendations to increase HR analytics adoption and to increase its effectiveness.
- Provide tailored Training and Support: Organizations should offer customized training to meet sector-specific needs. For complex sectors like manufacturing, extensive training is crucial.
- Enhance Tool Availability: Improve access to advanced analytics tools in resource-limited sectors, possibly through cost-effective solutions or phased upgrades.
- Strengthen Organizational Support: Cultivate a supportive culture where leadership promotes data-driven practices and provides necessary resources.
- Leverage Performance Expectancy: Highlight the benefits of HR analytics to drive adoption by linking it to performance improvements.
- Address Social Influence: Understand and manage peer expectations to align analytics practices with industry standards.
- Closing Words
Though HR analytics is very useful, HR data is very complex. There is also a need to consider both quantitative and qualitative data to arrive at more correct solutions to the problems you face. There is a need to apply the right kind of analytics to the right kind of data.
Added to these challenges is the problem of lack of adoption of HR analytics in India, which leads to a lot of data, but no real use of it, a lack of knowledge on how to use the analytics and analytics tools effectively, and a lack of buy-in from the leadership to improve the situation, especially in smaller companies. To improve the situation, there is a need for a clearer plan for adoption, allocation of resources for training, and guidance and support from the leadership.